1
2
3
4
5
6
7
8
9
10
11
12
13
14
15
16
17
18
19
20
21
22
23
24
25
26
27
28
29
30
31
32
33
34
35
36
37
38
39
40
41
42
43
44
45
46
47
48
49
50
51
52
53
54
55
56
57
58
59
60
61
62
63
64
65
66
67
68
69
70
71
72
73
74
75
76
77
78
79
80
81
82
83
84
85
86
87
88
89
90
91
92
93
94
95
96
97
98
99
100
101
102
103
104
105
106
107
108
109
110
111
112
113
114
115
116
117
118
119
120
121
122
123
124
125
126
127
128
129
130
131
132
133
134
135
136
137
138
139
140
141
142
143
144
145
146
147
148
149
150
151
152
153
154
155
156
157
158
159
160
161
162
163
164
165
166
167
168
169
170
171
172
173
174
175
176
177
178
179
180
181
182
183
184
185
186
187
188
189
190
191
192
193
194
195
196
197
198
199
200
201
202
203
204
205
206
207
208
209
210
211
212
213
214
215
216
217
218
219
220
221
222
223
224
225
226
227
228
229
230
231
232
233
234
235
236
237
238
239
240
241
242
243
244
|
from typing import Union, List
import torch
import torch.nn as nn
import torch.nn.functional as F
class ConvBNReLU(nn.Module):
"""这三个经常一起使用"""
def __init__(self, in_ch: int, out_ch: int, kernel_size: int = 3, dilation: int = 1): # dilation>1代表是膨胀卷积
super().__init__()
padding = kernel_size // 2 if dilation == 1 else dilation
self.conv = nn.Conv2d(in_ch, out_ch, kernel_size, padding=padding, dilation=dilation, bias=False)
self.bn = nn.BatchNorm2d(out_ch)
self.relu = nn.ReLU(inplace=True)
def forward(self, x: torch.Tensor) -> torch.Tensor:
return self.relu(self.bn(self.conv(x)))
class DownConvBNReLU(ConvBNReLU):
"""Encoder部分的下采样,卷积,BN,ReLU"""
def __init__(self, in_ch: int, out_ch: int, kernel_size: int = 3, dilation: int = 1, flag: bool = True):
super().__init__(in_ch, out_ch, kernel_size, dilation)
self.down_flag = flag # 是否启用下采样。默认为True
def forward(self, x: torch.Tensor) -> torch.Tensor:
if self.down_flag:
x = F.max_pool2d(x, kernel_size=2, stride=2, ceil_mode=True) # 两倍下采样
return self.relu(self.bn(self.conv(x)))
class UpConvBNReLU(ConvBNReLU):
"""Decoder部分的上采样,卷积,BN,ReLU"""
def __init__(self, in_ch: int, out_ch: int, kernel_size: int = 3, dilation: int = 1, flag: bool = True):
super().__init__(in_ch, out_ch, kernel_size, dilation)
self.up_flag = flag
def forward(self, x1: torch.Tensor, x2: torch.Tensor) -> torch.Tensor: # 两个tensor进行拼接
if self.up_flag:
x1 = F.interpolate(x1, size=x2.shape[2:], mode='bilinear', align_corners=False) # 这里采用双线性插值。x2是encoder输出的,.shape[2:]对应
return self.relu(self.bn(self.conv(torch.cat([x1, x2], dim=1))))
class RSU(nn.Module):
"""通用的RSU模块
height:深度,传入不同的height来实现RSU7..6..5...4
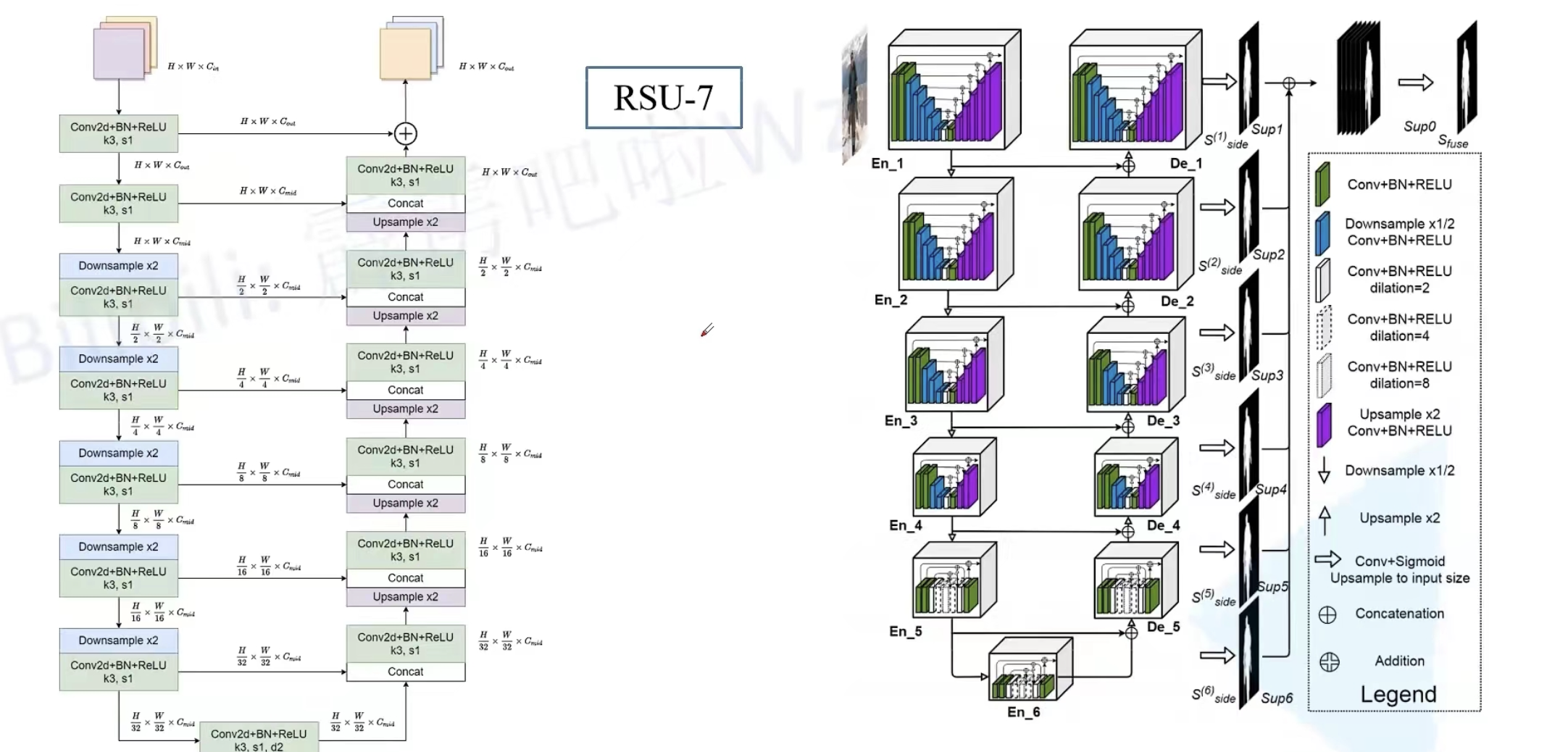
"""
def __init__(self, height: int, in_ch: int, mid_ch: int, out_ch: int):
super().__init__()
assert height >= 2
self.conv_in = ConvBNReLU(in_ch, out_ch)
encode_list = [DownConvBNReLU(out_ch, mid_ch, flag=False)] # 最开始对应的是encoder中比较特殊的简单的那个模块(左上角)
decode_list = [UpConvBNReLU(mid_ch * 2, mid_ch, flag=False)] # 最开始对应的是decoder中比较特殊的简单的那个模块(右下角)
for i in range(height - 2): # height - 2就是其中有上下采样的模块的数量
encode_list.append(DownConvBNReLU(mid_ch, mid_ch))
decode_list.append(UpConvBNReLU(mid_ch * 2, mid_ch if i < height - 3 else out_ch)) # 除了最后一个模块之外的,其他都是mid_ch
encode_list.append(ConvBNReLU(mid_ch, mid_ch, dilation=2)) # 最后添加一个(最底下的)
self.encode_modules = nn.ModuleList(encode_list)
self.decode_modules = nn.ModuleList(decode_list)
def forward(self, x: torch.Tensor) -> torch.Tensor:
x_in = self.conv_in(x)
x = x_in
encode_outputs = [] # 用来收集每个encoder的输出
for m in self.encode_modules:
x = m(x) # 输入的x依次放进去
encode_outputs.append(x)
x = encode_outputs.pop() # 最后一个encoder的膨胀卷积的输出弹出来
for m in self.decode_modules:
x2 = encode_outputs.pop()
x = m(x, x2) # 两个tensor传入到decoder中得到一个输出
return x + x_in # 加上最开始模块的输出
class RSU4F(nn.Module):
"""在RSU4的基础上将所有上下采样替换为膨胀卷积
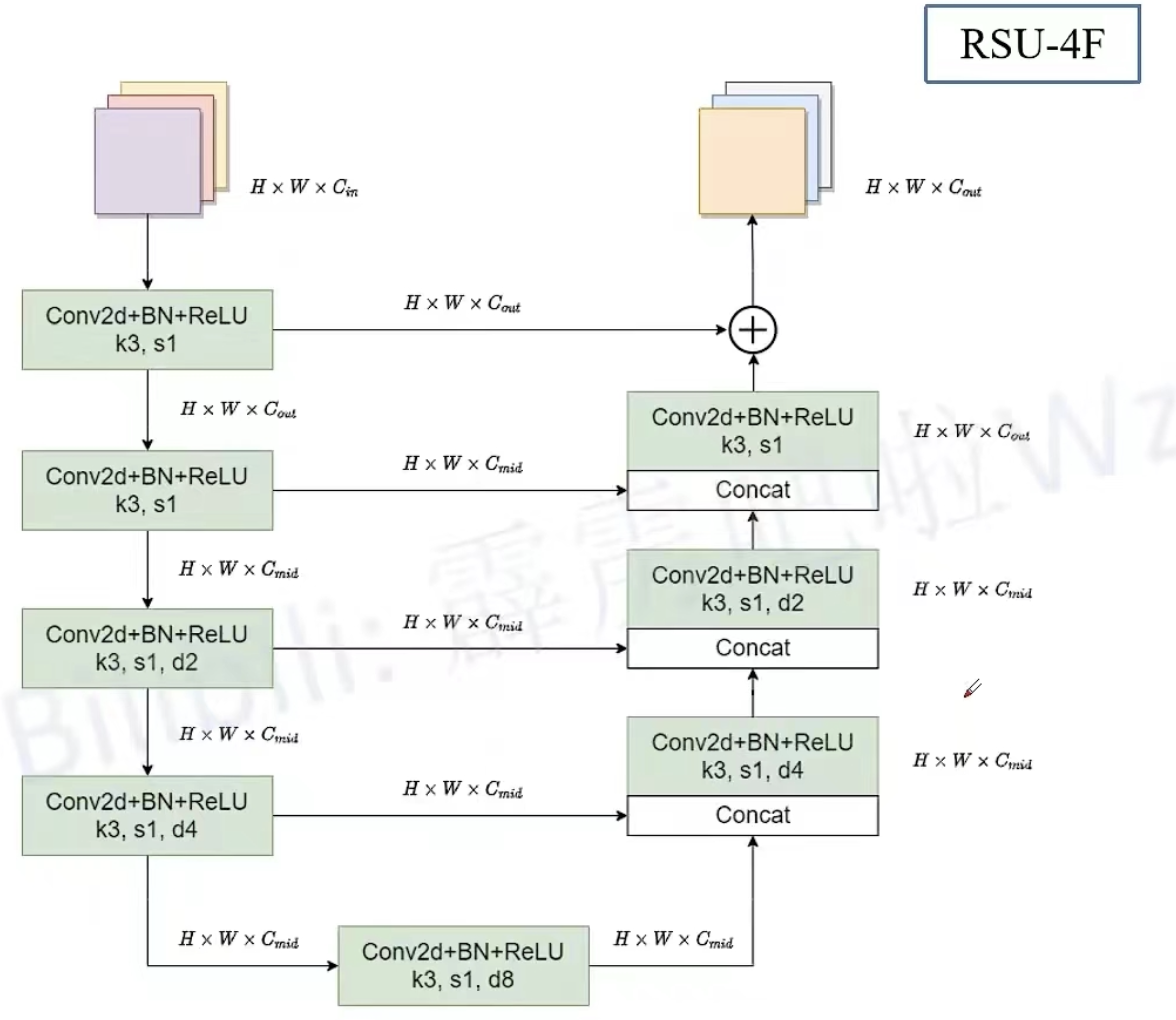
"""
def __init__(self, in_ch: int, mid_ch: int, out_ch: int):
super().__init__()
self.conv_in = ConvBNReLU(in_ch, out_ch)
self.encode_modules = nn.ModuleList([ConvBNReLU(out_ch, mid_ch),
ConvBNReLU(mid_ch, mid_ch, dilation=2),
ConvBNReLU(mid_ch, mid_ch, dilation=4),
ConvBNReLU(mid_ch, mid_ch, dilation=8)])
self.decode_modules = nn.ModuleList([ConvBNReLU(mid_ch * 2, mid_ch, dilation=4),
ConvBNReLU(mid_ch * 2, mid_ch, dilation=2),
ConvBNReLU(mid_ch * 2, out_ch)])
def forward(self, x: torch.Tensor) -> torch.Tensor:
x_in = self.conv_in(x)
x = x_in
encode_outputs = []
for m in self.encode_modules:
x = m(x)
encode_outputs.append(x)
x = encode_outputs.pop()
for m in self.decode_modules:
x2 = encode_outputs.pop()
x = m(torch.cat([x, x2], dim=1))
return x + x_in
class U2Net(nn.Module):
"""最终的网络"""
def __init__(self, cfg: dict, out_ch: int = 1): # 这里显著性目标检测,所以out_ch=1
super().__init__()
assert "encode" in cfg
assert "decode" in cfg
self.encode_num = len(cfg["encode"])
encode_list = []
side_list = []
for c in cfg["encode"]:
# c: [height, in_ch, mid_ch, out_ch, RSU4F, side]
assert len(c) == 6
encode_list.append(RSU(*c[:4]) if c[4] is False else RSU4F(*c[1:4]))
if c[5] is True:
side_list.append(nn.Conv2d(c[3], out_ch, kernel_size=3, padding=1))
self.encode_modules = nn.ModuleList(encode_list)
decode_list = []
for c in cfg["decode"]:
# c: [height, in_ch, mid_ch, out_ch, RSU4F, side]
assert len(c) == 6
decode_list.append(RSU(*c[:4]) if c[4] is False else RSU4F(*c[1:4]))
if c[5] is True:
side_list.append(nn.Conv2d(c[3], out_ch, kernel_size=3, padding=1))
self.decode_modules = nn.ModuleList(decode_list)
self.side_modules = nn.ModuleList(side_list)
self.out_conv = nn.Conv2d(self.encode_num * out_ch, out_ch, kernel_size=1) # 1*1的卷积层融合不同尺度的信息
def forward(self, x: torch.Tensor) -> Union[torch.Tensor, List[torch.Tensor]]:
_, _, h, w = x.shape
# collect encode outputs
encode_outputs = []
for i, m in enumerate(self.encode_modules):
x = m(x)
encode_outputs.append(x)
if i != self.encode_num - 1:
x = F.max_pool2d(x, kernel_size=2, stride=2, ceil_mode=True)
# collect decode outputs
x = encode_outputs.pop()
decode_outputs = [x]
for m in self.decode_modules:
x2 = encode_outputs.pop()
x = F.interpolate(x, size=x2.shape[2:], mode='bilinear', align_corners=False)
x = m(torch.concat([x, x2], dim=1))
decode_outputs.insert(0, x)
# collect side outputs
side_outputs = []
for m in self.side_modules:
x = decode_outputs.pop()
x = F.interpolate(m(x), size=[h, w], mode='bilinear', align_corners=False)
side_outputs.insert(0, x)
x = self.out_conv(torch.concat(side_outputs, dim=1))
if self.training:
# do not use torch.sigmoid for amp safe
return [x] + side_outputs
else:
return torch.sigmoid(x)
def u2net_full(out_ch: int = 1):
cfg = {
# height, in_ch, mid_ch, out_ch, RSU4F, side
"encode": [[7, 3, 32, 64, False, False], # En1
[6, 64, 32, 128, False, False], # En2
[5, 128, 64, 256, False, False], # En3
[4, 256, 128, 512, False, False], # En4
[4, 512, 256, 512, True, False], # En5
[4, 512, 256, 512, True, True]], # En6
# height, in_ch, mid_ch, out_ch, RSU4F, side
"decode": [[4, 1024, 256, 512, True, True], # De5
[4, 1024, 128, 256, False, True], # De4
[5, 512, 64, 128, False, True], # De3
[6, 256, 32, 64, False, True], # De2
[7, 128, 16, 64, False, True]] # De1
}
return U2Net(cfg, out_ch)
def u2net_lite(out_ch: int = 1):
cfg = {
# height, in_ch, mid_ch, out_ch, RSU4F, side
"encode": [[7, 3, 16, 64, False, False], # En1
[6, 64, 16, 64, False, False], # En2
[5, 64, 16, 64, False, False], # En3
[4, 64, 16, 64, False, False], # En4
[4, 64, 16, 64, True, False], # En5
[4, 64, 16, 64, True, True]], # En6
# height, in_ch, mid_ch, out_ch, RSU4F, side
"decode": [[4, 128, 16, 64, True, True], # De5
[4, 128, 16, 64, False, True], # De4
[5, 128, 16, 64, False, True], # De3
[6, 128, 16, 64, False, True], # De2
[7, 128, 16, 64, False, True]] # De1
}
return U2Net(cfg, out_ch)
def convert_onnx(m, save_path):
m.eval()
x = torch.rand(1, 3, 288, 288, requires_grad=True)
# export the model
torch.onnx.export(m, # model being run
x, # model input (or a tuple for multiple inputs)
save_path, # where to save the model (can be a file or file-like object)
export_params=True,
opset_version=11)
if __name__ == '__main__':
# n_m = RSU(height=7, in_ch=3, mid_ch=12, out_ch=3)
# convert_onnx(n_m, "RSU7.onnx")
#
# n_m = RSU4F(in_ch=3, mid_ch=12, out_ch=3)
# convert_onnx(n_m, "RSU4F.onnx")
u2net = u2net_full()
convert_onnx(u2net, "u2net_full.onnx")
|